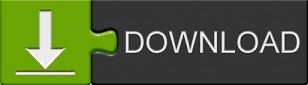
The Titanic tragedy is the most well-known maritime disaster of modern history, and the Titanic dataset is a widely used and first-rate example for the teaching of mono-method statistical explanation.

The goal of this paper is to show that the case of the Titanic, and the question of who survived and for what reasons, can be seen as such an example. While several scholars have argued that mixed methods may help to improve sociological explanations, there is a lack of highly visible examples that show the added value of this methodology. The question of how and with what methods the social sciences should explain phenomena is fiercely contested. Findings of this study showed that some passenger groups were more likely to survive than others, with respect to certain demographic characteristic and whether the passenger was traveling in the first, second or third class. Secondary data was used as the main data collection tool and it was analyzed by fitting a logistic regression model using a statistical package, SPSS. The main aim of this research is to identify the Impact of gender, passenger class, Accompany, age on a person?s likelihood of surviving the shipwreck. However there are many reasons that the shipwreck led to such loss of life and there was some elements of luck involved in surviving the sinking as some groups of people were more likely to survive than others. This sensational tragedy shocked the international community and motivated the adoption of better maritime safety regulations. On April 15, 1912, during her maiden voyage, the Titanic sank after colliding with an iceberg, killing 1,502 of the 2,228 passengers and crew. The scatter plot shows that the dataset cannot be separated into positive and negative examples by a straight-line through the plot.The sinking of Titanic is one of the most infamous shipwrecks in history. Plot the data creating a figure, where the axes are the two test scores, and the positive ( $y=1$, accepted) and negative ( $y=0$, rejected) examples are shown with different markers. data2 = pd.read_csv('ex2data2.txt', header=None, names=) From these two tests, it will be determined whether the microchips should be accepted or rejected.
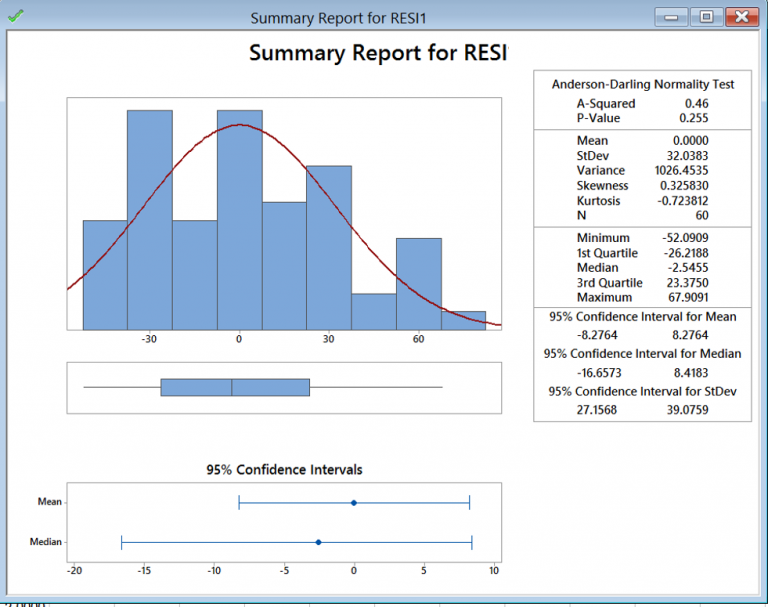
There are test results for some microchips on two different tests. A dataset of test results on past microchips will be used to build a logistic regression model. During QA, each microchip goes through various tests to ensure it is functioning correctly. $J(\theta)=\frac%'.format(100 * correct / total))Ī regularized logistic regression will be implemented to predict whether microchips from a fabrication plant passes quality assurance (QA). The cost function in logistic regression is: Implement the cost function and gradient for logistic regression.
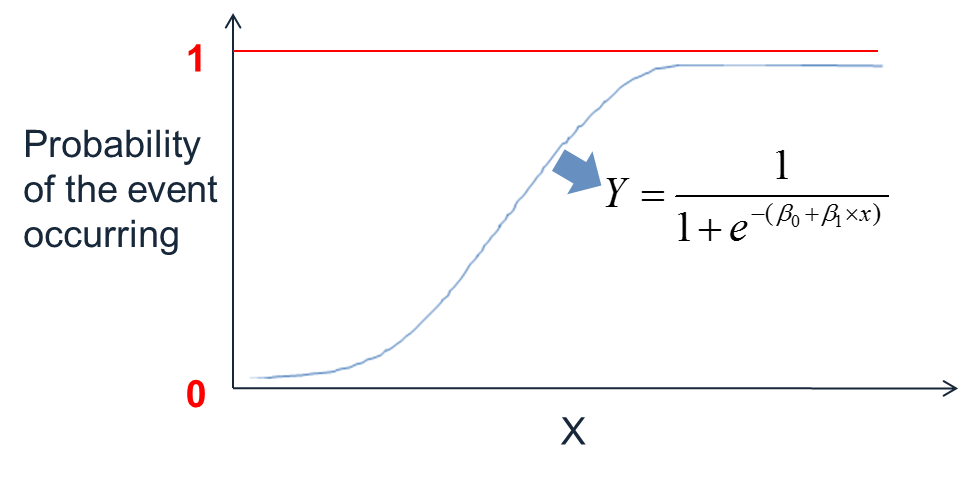
Print('Evaluate sigmoid(0) =', sigmoid(0)) For a matrix, the function should perform the sigmoid function on every element.
#LOGISTIC REGRESSION JMP CODE#
The code should also work with vectors and matrices. Evaluating sigmoid(0) should give exactly 0.5. For large positive values of $x$, the sigmoid should be close to 1, while for large negative values, the sigmoid should be close to 0. The first step is to implement the sigmoid function. Where function g is the sigmoid function.
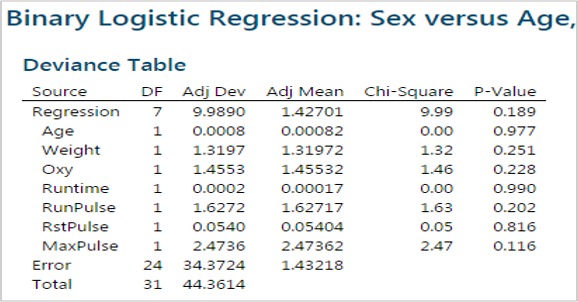
The logistic regression hypothesis is defined as: Plt.title('Scatter plot of training data') Plot the data creating a figure, where the axes are the two exam scores, and the positive and negative examples are shown with different markers.
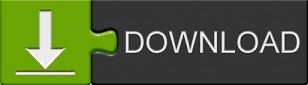